Artificial intelligence (AI) has been transforming the world of business for decades, enabling new levels of automation, efficiency, and intelligence. However, most AI applications today are based on analyzing existing data and making predictions or recommendations based on predefined rules or models. This type of AI is known as analytical AI, and it has limitations in terms of creativity, flexibility, and scalability.
A new wave of AI is emerging that can go beyond analysis and generate new, realistic, and diverse content from scratch or based on existing data. This type of AI is known as generative AI, and it has the potential to revolutionize various industries and domains by unlocking new possibilities for innovation, personalization, and optimization.

What is Generative AI and how does it work?
Generative AI is a set of algorithms, capable of generating seemingly new, realistic content—such as text, images, or audio—from the training data. The most powerful generative AI algorithms are built on top of foundation models that are trained on a vast quantity of unlabeled data in a self-supervised way to identify underlying patterns for a wide range of tasks. For example, GPT-3.5, a foundation model trained on large volumes of text, can be adapted for answering questions, text summarization, or sentiment analysis. DALL-E, a multimodal (text-to-image) foundation model, can be adapted to create images, expand images beyond their original size, or create variations of existing paintings.
Generative AI uses a number of techniques that continue to evolve. One of the most popular techniques is Generative Adversarial Networks (GANs), which consist of two competing neural networks: a generator and a discriminator. The generator tries to create realistic outputs that can fool the discriminator, while the discriminator tries to distinguish between real and fake outputs. The process continues until the generator produces outputs that are indistinguishable from the real ones.
Another technique is Variational AutoEncoders (VAEs), which are neural networks that can learn to compress data into a lower-dimensional representation and then reconstruct it with minimal loss of information. VAEs can also generate new data by sampling from the learned representation space, which captures the essential features of the data.
What are the benefits and applications of Generative AI?
Generative AI can bring unprecedented speed and creativity to areas like design, research, and copy generation. It can also take business process automation to a transformative new level, catalyzing a new era of efficiency in both the back and front offices. The capabilities that generative AI supports can be summarized into three categories:
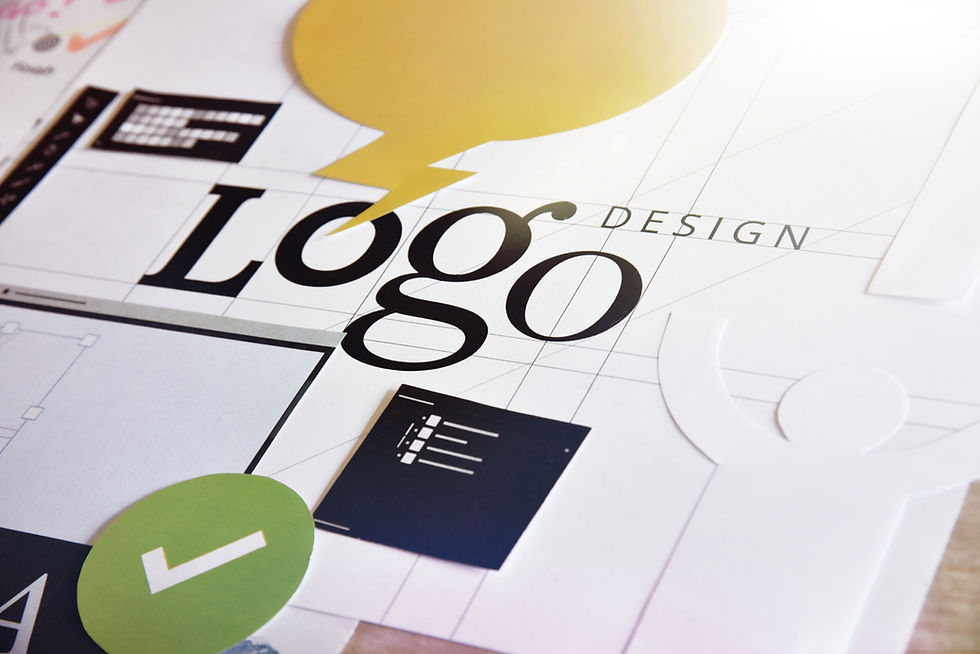
- Generating Content and Ideas
Generative AI can create new, unique outputs across a range of modalities, such as a video advertisement or even a new protein with antimicrobial properties. For example, generative AI can help designers create logos, websites, or product prototypes by providing them with multiple options based on their preferences or feedback. Generative AI can also help researchers discover new drugs, materials, or molecules by generating novel candidates that meet certain criteria or specifications.

- Improving Efficiency
Generative AI can accelerate manual or repetitive tasks, such as writing emails, coding, or summarizing large documents. For example, generative AI can help salespeople compose personalized and engaging emails to potential customers by generating relevant and catchy subject lines, greetings, and calls to action. Generative AI can also help developers write code faster and with fewer errors by generating snippets of code based on natural language descriptions or examples.
- Personalizing Experiences
Generative AI can create content and information tailored to a specific audience, such as chatbots for a personalized customer experience or targeted advertisements based on patterns in a specific customer's behavior. For example, generative AI can help marketers create dynamic and customized ads that adapt to the context and preferences of each viewer, such as changing the color, style, or message of the ad. Generative AI can also help customer service agents provide better and faster support by generating natural and empathetic responses to customer queries or complaints.
What are the challenges and risks of Generative AI?
Generative AI is not without challenges and risks, both technical and ethical. Some of the main challenges and risks are:
- Data Quality and Availability. Generative AI requires large amounts of high-quality and diverse data to train the models and generate realistic and relevant outputs. However, data can be scarce, noisy, biased, or incomplete, which can affect the performance and accuracy of the models and the outputs. Moreover, data can be sensitive, proprietary, or regulated, which can pose challenges for data access, sharing, and protection.
- Model Complexity and Interpretability
Generative AI models are often very complex and opaque, making it difficult to understand how they work and why they generate certain outputs. This can lead to challenges for model validation, debugging, and improvement, as well as for explaining and justifying the outputs to the stakeholders and users. Moreover, model complexity can also increase the computational and energy costs of training and running the models, which can limit the scalability and sustainability of generative AI applications.
- Ethical and Social Implications
Generative AI can have significant ethical and social implications, such as privacy, security, accountability, and fairness. For example, Generative AI can create realistic and convincing fake content, such as deepfakes, that can be used for malicious purposes, such as fraud, deception, or manipulation. Generative AI can also generate biased or harmful content, such as hate speech, misinformation, or plagiarism, that can affect the reputation, trust, and well-being of individuals and organizations. Furthermore, Generative AI can raise questions about the ownership, authorship, and rights of the generated content, as well as the impact of Generative AI on human creativity, agency, and dignity.
How can businesses prepare for and leverage Generative AI?
Generative AI is a powerful and disruptive technology that can create new opportunities and challenges for businesses across industries and domains. To prepare for and leverage Generative AI, businesses need to:
- Define a clear and compelling Generative AI strategy
Businesses need to identify and prioritize the use cases and applications of Generative AI that can create the most value and impact for their goals and objectives. Businesses also need to assess and align their capabilities and resources, such as data, talent, infrastructure, and governance, to support and enable their Generative AI strategy.
- Develop a responsible and trustworthy Generative AI practice
Businesses need to adopt and implement best practices and standards for developing, deploying, and using Generative AI in a responsible and trustworthy manner. Businesses also need to monitor and evaluate the performance and outcomes of Generative AI, as well as the risks and issues that may arise, and take appropriate actions to mitigate and resolve them. Businesses also need to engage and communicate with their stakeholders and users, such as customers, employees, partners, and regulators, to ensure transparency, accountability, and consent for Generative AI.
- Foster a culture of innovation and collaboration for Generative AI
Businesses need to cultivate a culture of innovation and collaboration that encourages and supports the exploration and experimentation of generative AI. Businesses also need to foster a culture of learning and adaptation that enables the continuous improvement and optimization of generative AI. Businesses also need to foster a culture of co-creation and co-evolution that leverages the complementary strengths and skills of humans and machines for generative AI.
Conclusion
Generative AI is a game-changing technology that can unlock new possibilities for business innovation, efficiency, and personalization. However, Generative AI also poses significant challenges and risks that require careful and responsible management. Businesses that can harness the potential of Generative AI while addressing its pitfalls will gain a competitive edge and a sustainable advantage in the future.
Source
(1) Generative AI is a toddler — don’t let it run your business. https://venturebeat.com/ai/generative-ai-is-a-toddler-dont-let-it-run-your-business/.
(2) Generative AI: UK Business Leaders Face Investment Challenges as Everyone Claims to Be an Expert. https://www.techrepublic.com/article/generative-ai-uk-business-investment-challenges/.
(3) A growing number of businesses are now going all-in on generative AI. https://www.msn.com/en-us/money/companies/a-growing-number-of-businesses-are-now-going-all-in-on-generative-ai/ar-AA1kpvJg.
(4) What is Generative AI and How Does it Impact Businesses? | BCG. https://www.bcg.com/capabilities/artificial-intelligence/generative-ai.
(5) Generative AI: What Is It, Tools, Models, Applications and Use Cases. https://www.gartner.com/en/topics/generative-ai.
(6) What is Generative AI & Why is It Important? | Accenture. https://www.accenture.com/in-en/insights/generative-ai.